
Joshua Denny, M.D., M.S.
Adjunct Investigator
Precision Genomics Section
Head
Precision Health Informatics Section
Education
B.S. Vanderbilt University
M.D. Vanderbilt University
M.S. Biomedical Informatics, Vanderbilt University
Precision Health Informatics Section Staff

Tracey Ferrara, Ph.D.
- Lab Manager
- Precision Health Informatics Section
Staff Clinicians
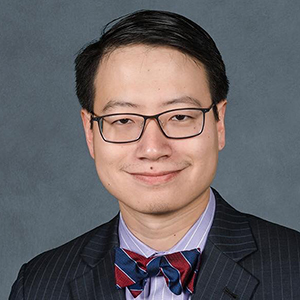
Huan Mo, M.D., M.S.
- Director
- Cohort Analytics Core
Staff Scientists
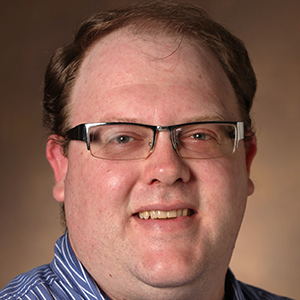
Jacob Keaton, Ph.D.
- Staff Scientist
- Precision Health Informatics Section

Chenjie Zeng, Ph.D., M.P.H.
- Staff Scientist
- Precision Health Informatics Section
Postdoctoral Fellows
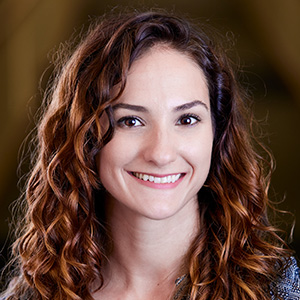
Slavina Goleva, Ph.D.
- Postdoctoral Fellow
- Precision Health Informatics Section

Ariel Williams, M.S., Ph.D.
- Postdoctoral Fellow
- Precision Health Informatics Section
Clinical Fellows

Bennett Waxse, M.D., Ph.D.
- Clinical Fellow
- Precision Health Informatics Section
Bioinformaticians
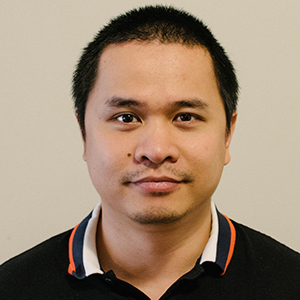
Tam Tran, M.S.
- Bioinformatician
- Cohorts Data Analytics Core
Postbaccalaureate Fellows
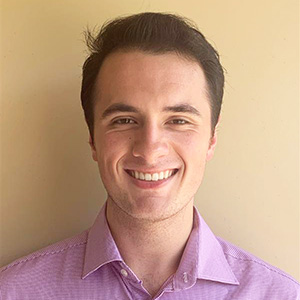
Peter J. Sauer, B.S.
- Postbaccalaureate Fellow
- Precision Health Informatics Section
Student IRTAs
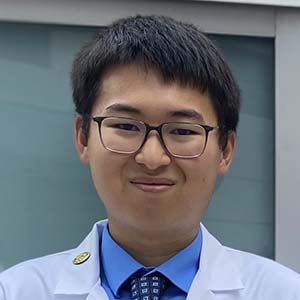
James Yang
- Student IRTA
- Precision Health Informatics Section
Special Volunteers

Anav Babbar
- Special Volunteer
- Precision Health Informatics Section
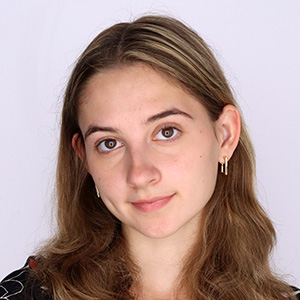
Molly Goldwasser
- Special Volunteer
- Precision Health Informatics Section

David Schlueter, Ph.D.
- Special Volunteer
- Precision Health Informatics Section

Onajia Stubblefield, M.S.
- Special Volunteer
- Precision Health Informatics Section
Last updated: December 14, 2023