The Big Picture
- The genomics field continues to expand the use of computational methods such as artificial intelligence and machine learning to improve our understanding of hidden patterns in large and complex genomics data sets from basic and clinical research projects.
- Machine learning analyses could benefit disease research and genomic tools like CRISPR.
- NHGRI is identifying and shaping its unique role in the convergence of genomic and machine learning research.
What is artificial intelligence?
There are many definitions for artificial intelligence (AI). One such definition for AI is “AI is a science and a set of computational technologies that are inspired by — but typically operate quite differently from — the ways people use their nervous systems and bodies to sense, learn, reason, and take action.” AI can be created as software or tools that are able to mimic human intelligence in certain contexts or even exceed it in others.
To be able to build AI, scientists need large, well-explained datasets that first help them understand the techniques and processes used by humans to analyze and interpret complex scenarios. The field of AI is a dynamic one, and researchers are consistently developing new techniques and tools.
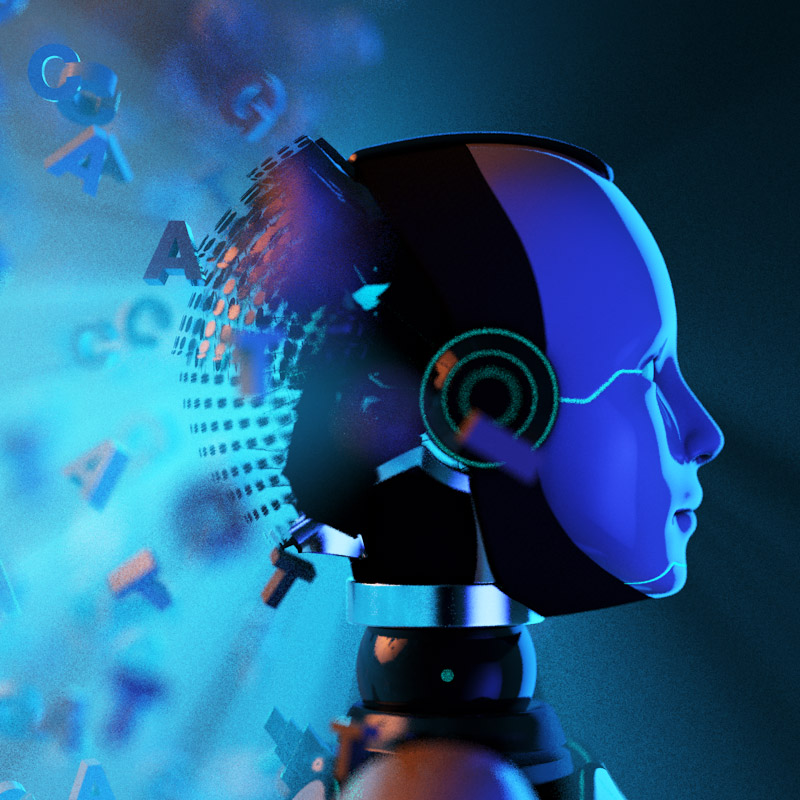
What are machine learning and deep learning?
Machine learning (ML) and deep learning are fields of study frequently mentioned in the context of AI. Both kinds of learning are subfields of AI. Machine learning is a process by which machines can be given the capability to learn about a given dataset without being explicitly programmed on what to learn.
Machines can usually learn in either a supervised or unsupervised manner. Under supervised learning, scientists provide machines with separate training and test data sets. The training data has defined categories (e.g., people with coronary heart disease and those without) that the machine can use to infer hidden qualities of the data and distinguish the categories from each other. It is then able to use this knowledge to work on the test data and make informed predictions (e.g., which people in a population are likely to develop coronary heart disease).
In an unsupervised learning setting, machines can recognize patterns in large datasets and make predictions about the real world without requiring any additional help from humans.
When machines can learn in an unsupervised manner, they are considered to be learning “deeply.” Deep learning is a relatively modern technique used to implement machine learning. A deep learning algorithm takes a dataset and finds patterns and critical information by imitating how a human brain’s neurons interact with each other. The algorithms are artificial neural networks — a computing system that simulates the brain’s ability to weigh the importance of some data versus others, and handle bias.
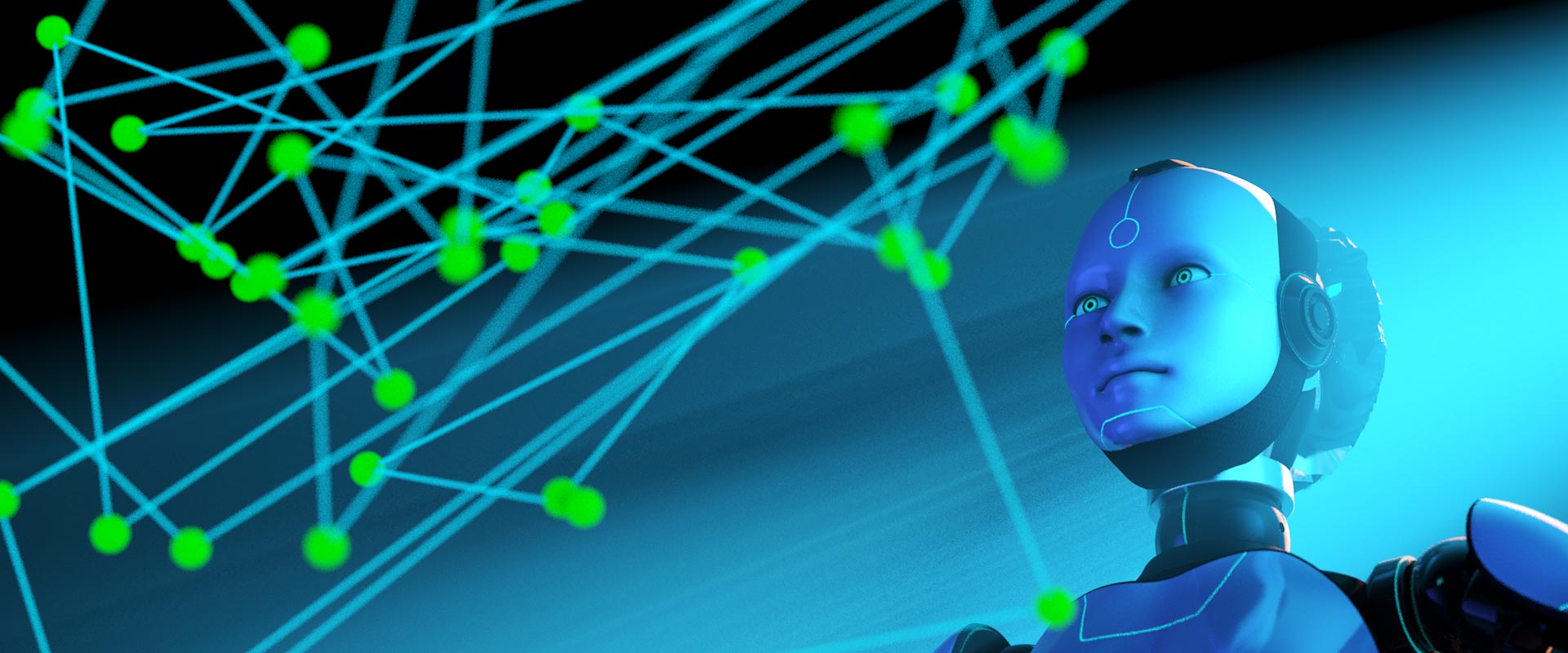
Why is there a need for AI/ML in genomics?
As of 2021, 20 years have passed since the landmark completion of the draft human genome sequence. This milestone has led to the generation of an extraordinary amount of genomic data. Estimates predict that genomics research will generate between 2 and 40 exabytes of data within the next decade.
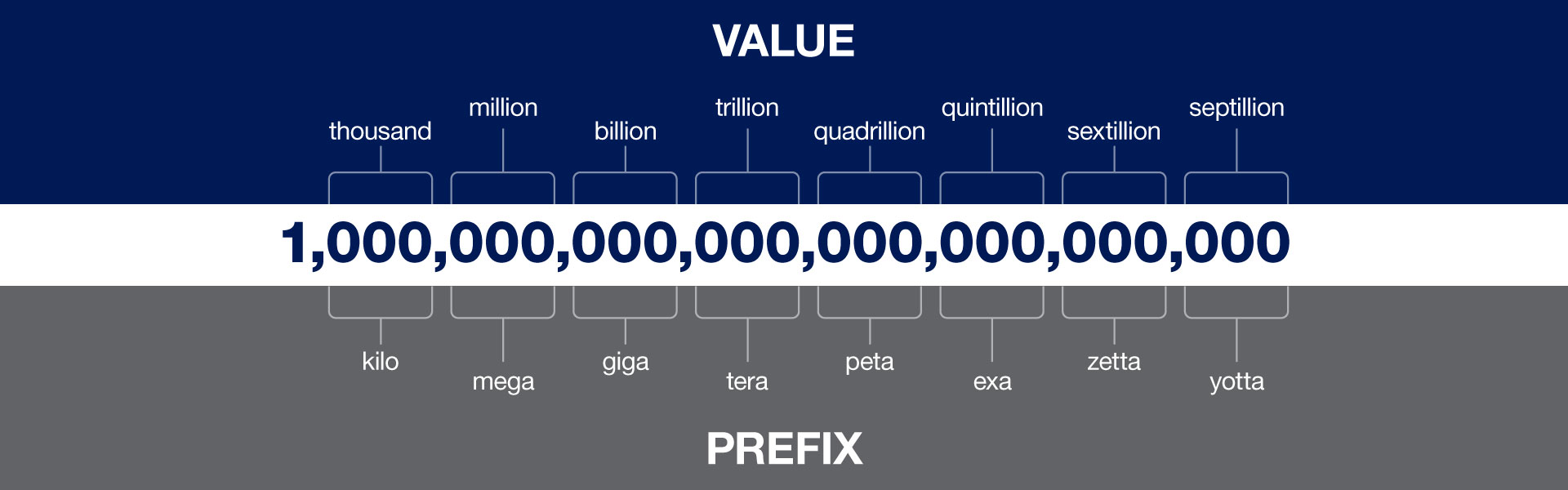
DNA sequencing and other biological techniques will continue to increase the number and complexity of such data sets. This is why genomics researchers need AI/ML-based computational tools that can handle, extract and interpret the valuable information hidden within this large trove of data.
- Thousand: Kilo
- Million: Mega
- Billion: Giga
- Trillion: Tera
- Quadrillion: Peta
- Quintillion: Exa
- Sextillion: Zetta
- Septillion: Yotta
What are some ways in which AI/ML are being used in genomics?
Although the use of AI/ML tools in genomics is still at an early stage, researchers have already benefited from developing programs that assist in specific ways.
Some examples include:
- Examining people’s faces with facial analysis AI programs to accurately identify genetic disorders.
- Using machine learning techniques to identify the primary kind of cancer from a liquid biopsy.
- Predicting how a certain kind of cancer will progress in a patient.
- Identifying disease-causing genomic variants compared to benign variants using machine learning.
- Using deep learning to improve the function of gene editing tools such as CRISPR.
These are just a few ways by which AI/ML methods are helping predict and identify hidden patterns in genomic data. Scientists are also using AI/ML to predict future variations in the genomes of the influenza and SARS-CoV-2 viruses to assist public health efforts.

Last updated: January 12, 2022